Healthcare AI and HIPAA privacy concerns: Everything you need to know
Healthcare AI has enormous potential to benefit practices — and to violate HIPAA. Here’s what you need to know to stay in compliance.
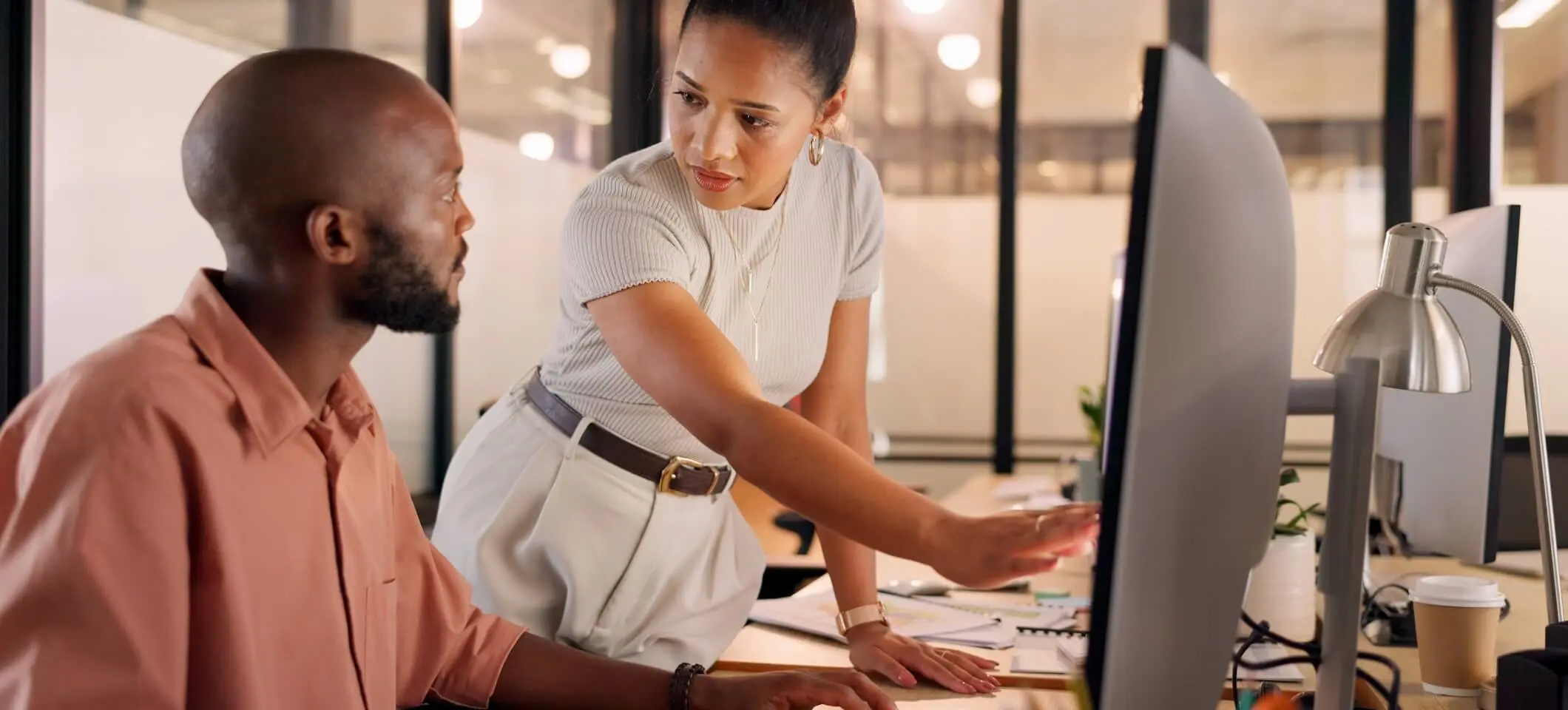
Most Popular
At a Glance
- AI in healthcare raises concerns about data security and patient privacy.
- Compliance with HIPAA and GDPR protects sensitive health information.
- Transparent AI policies build patient trust and regulatory compliance.
Artificial intelligence (AI) in healthcare leverages computer systems to interpret complex medical data, make predictions based on patterns, and assist in decision-making processes, often at a speed and accuracy that exceeds human capabilities.
This technology encompasses a range of methods, including machine learning algorithms and predictive analytics. It has the potential to significantly reshape patient care, operational efficiencies, and clinical outcomes.
That said, there are many important considerations that accompany the introduction of healthcare AI. Compliance with the Health Insurance Portability and Accountability Act (HIPAA) is one of the most complex.
HIPAA, a critical regulatory framework in the U.S. healthcare system, sets the standard for protecting sensitive patient data. As healthcare providers increasingly adopt AI tools, they must balance 2 key goals. On the one hand, providers can leverage this innovative technology to improve patient care. On the other hand, they must ensure the confidentiality, integrity, and availability of patient data as mandated by HIPAA.
Read on to learn about AI’s impact on HIPAA and how it might affect your practice.
What are HIPAA-covered entities?
HIPAA compliance is required only for certain types of organizations. Understanding HIPAA's impact on AI begins with identifying the entities that are covered by HIPAA.
Coverage under HIPAA is determined by specific activities, not merely professional roles. For example, a physician becomes a HIPAA-covered entity not simply because of their medical profession, but because they engage in activities like billing insurance.
Similarly, healthcare providers, insurance companies, and clearinghouses become HIPAA-covered when they conduct certain transactions that require HIPAA compliance.
These HIPAA-covered entities, along with their business associates (BAs), must comply with HIPAA, particularly when implementing AI in healthcare services. Therefore, a provider who does not bill insurance, such as a dermatologist who only takes direct payments, would not fall under HIPAA.
Use the HHS online tool to determine if your organization is a HIPAA-covered entity or BA and, consequently, must comply.
HIPAA-compliance and AI: Understanding the rules
The HIPAA privacy rule is pivotal in protecting patient information, especially when employing AI technologies. This rule governs the use and disclosure of protected health information (PHI). It allows specific exceptions for treatment, payment, healthcare operations, and certain research conditions.
“In AI healthcare applications, managing [protected health information] correctly is crucial.”
These issues, including how to handle PHI for advanced research in areas like cancer, predicting public health trends, or healthcare reimbursements, were not fully anticipated during HIPAA's initial development.
In AI healthcare applications, managing PHI correctly is crucial. This involves stripping personal identifiers from patient data (de-identification) and obtaining patient consent for data use, ensuring privacy and trust.
Key to this discussion is the concept of a “limited data set” under HIPAA. This set includes some identifiable elements like ZIP codes or dates related to medical care, but excludes direct identifiers like names or social security numbers. While not completely de-identified, this data can be used for research purposes under HIPAA, provided there's a data use agreement that meets HIPAA's standards.
For AI-driven research or operations, understanding the nuances of PHI usage, including limited data sets and de-identification, is critical.
Under HIPAA's de-identification safe harbor, 18 specific identifiers must be removed from the data. Furthermore, there must be no way to re-identify the de-identified information, either alone or in combination with other data. This safeguard is especially pertinent when handling data for AI purposes, as the potential for re-identification might exist depending on the AI application and the data it processes.
HIPAA privacy and healthcare AI: A use case
AI in radiology can assist in interpreting medical images, such as MRIs and CT scans, with greater accuracy and speed. The aim is to enhance diagnostic processes and improve patient outcomes.
To perform the task, the AI system requires access to a vast amount of patient medical images, which contain PHI. Before feeding medical images into the AI system, the hospital deploys a rigorous process to de-identify the data. This involves removing all direct and indirect identifiers to ensure that individual patients could not be linked to the images.
For cases where de-identification is not feasible, the hospital must seek explicit patient consent. To facilitate this, use clear consent forms that outline how you will use patient data in AI research. It’s essential to highlight the potential benefits as well as the measures to ensure data privacy.
Additionally, it's vital for healthcare professionals to receive training on HIPAA compliance within AI space. This includes understanding the 21st Century Cures Act, particularly its provisions on information blocking and how they intersect with HIPAA.
“Use clear consent forms that outline how you will use patient data in AI research.”
"Information blocking" refers to unreasonably limiting the availability, disclosure, or use of electronic health information (EHI). It undermines efforts to improve interoperability, data sharing, and patient access to their health data. This could include activities like healthcare providers or IT vendors deliberately preventing or discouraging the sharing or use of EHI for reasons other than privacy and security.
Healthcare professionals should be aware of what constitutes information blocking and ensure they do not restrict the lawful and necessary sharing of EHI.
Healthcare AI models: Machine learning and deep learning
Machine learning and deep learning are types of AI models commonly used in healthcare due to their versatility and effectiveness in a range of applications.
The machine learning model enables systems to learn from data, identify patterns, and make decisions with minimal human input.
In supervised learning, these systems train on labeled data, enabling them to predict outcomes or identify diseases by learning from past examples. For instance, they can analyze patient data to foresee health risks or outcomes, which significantly enhances personalized healthcare.
This method is especially potent in diagnosing diseases. Machine learning can detect subtle patterns in imaging data, such as MRI or CT scans, that might elude human observation.
Unsupervised learning deals with unlabeled data. It is adept at uncovering hidden patterns or data clusters without prior knowledge, which is invaluable in drug discovery. By analyzing complex biological and chemical datasets, unsupervised machine learning can identify potential therapeutic compounds or novel drug targets, accelerating the pace of pharmaceutical research.
Deep learning is a subset of machine learning that uses complex neural networks to simulate the human brain. It excels in processing large amounts of unstructured data, like images and sound. It is particularly effective for tasks such as analyzing dermatological images for skin conditions, enabling more accurate and faster diagnoses than traditional methods.
There’s also immense promise for the genetics and genomics space. Deep learning can process and interpret complex genetic data to identify patterns and mutations that may be linked to specific conditions. This application is revolutionizing the field by aiding in the prediction of genetic disorders, understanding gene expression, and facilitating personalized medicine based on an individual's genetic makeup.
By leveraging deep learning, researchers and clinicians can gain deeper insights into genetic factors in health and disease to introduce more targeted and effective treatments.
HIPAA privacy and healthcare AI: The risks
Data collection
To accurately classify or predict outcomes, both machine learning and deep learning models rely on the availability of huge datasets. The more data these models have, the more they can learn patterns, generalize to new situations, and understand complexity.
However, the need for substantial data in healthcare AI faces a significant challenge in the form of HIPAA privacy security concerns. Healthcare practices are naturally reluctant to share data, but doing so is necessary for machine learning and deep learning models to be effective.
“The need for substantial data in healthcare AI faces a significant challenge in the form of HIPAA privacy security concerns.”
The risk here is multifaceted. First, without sufficient data, these models may not reach their full potential in predictive accuracy. This can limit their effectiveness in clinical decision-making, research, and patient care.
Also, there's the risk of AI models imparting their inherent biases to healthcare professionals. These biases, once absorbed, can persist and influence medical judgments and patient interactions, even after the use of AI has ceased. This underscores the need to consider both the quantity and quality of data used in training healthcare AI systems, to mitigate the risk of perpetuating biases.
Finally, if healthcare organizations choose to share data, they must navigate HIPAA to avoid breaching patient confidentiality.
How to mitigate data collection risks
This includes de-identifying data, securing consent, and establishing robust data sharing agreements that comply with HIPAA.
Unless using a closed system, users must assume that any information they input could enter the public domain.
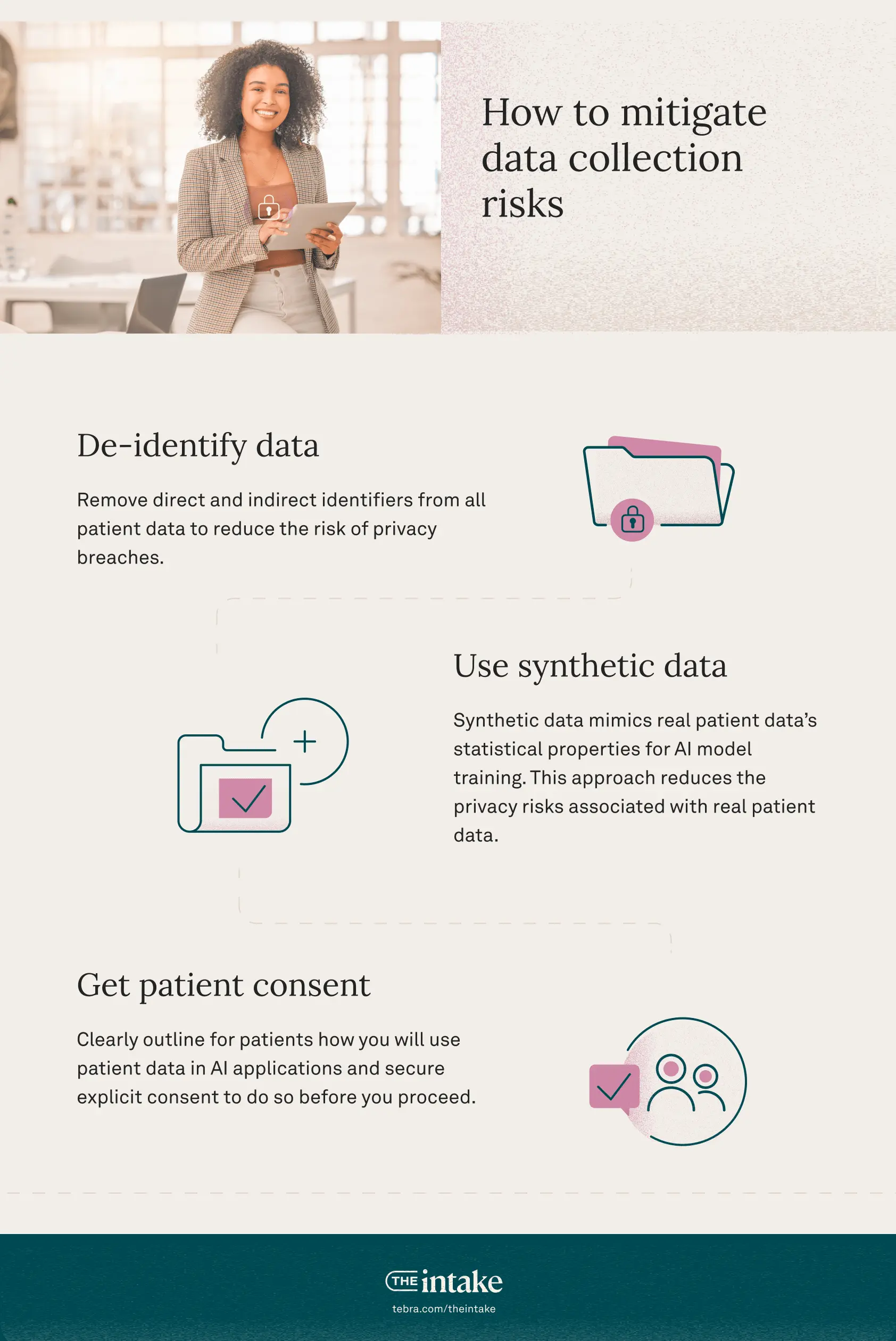
Security
AI systems in healthcare raise significant data security and privacy concerns.
The sensitivity and high value of health records make them a prime target for cyberattacks and data breaches.
In fact, New York has proposed a slew of new cybersecurity regulations for the state’s hospitals and plans to funnel $500 million from its fiscal year 2024 budget to help facilities upgrade their technology systems. Ensuring the confidentiality and integrity of medical records is not just a regulatory requirement but a crucial aspect of maintaining patient trust and safety.
“Healthcare organizations employing AI must implement robust security measures to protect against unauthorized access and data breaches.”
One emerging concern is the potential for people to confuse AI-driven systems for real health professionals. Because AI can seem very human-like, patients might end up sharing more personal health information than they intend, thinking they're talking to a person. This mistake could lead patients to unknowingly agree to give more information than they otherwise would.
AI models can process and analyze large amounts of data in non-transparent ways, leading to significant privacy concerns. This opacity in decision-making, particularly when AI models are used to assist in diagnosis and therapy, underscores the need for transparent communication and informed consent.
Another critical concern in addition to data security and privacy is bias in data handling and decision-making. Biases in AI systems, stemming from their design or the data on which they are trained, can lead to unequal or unfair treatment and privacy considerations for different patient groups.
How to mitigate security risks
Healthcare organizations employing AI must implement robust security measures to protect against unauthorized access and data breaches. This includes encryption, access controls, and regular security audits.
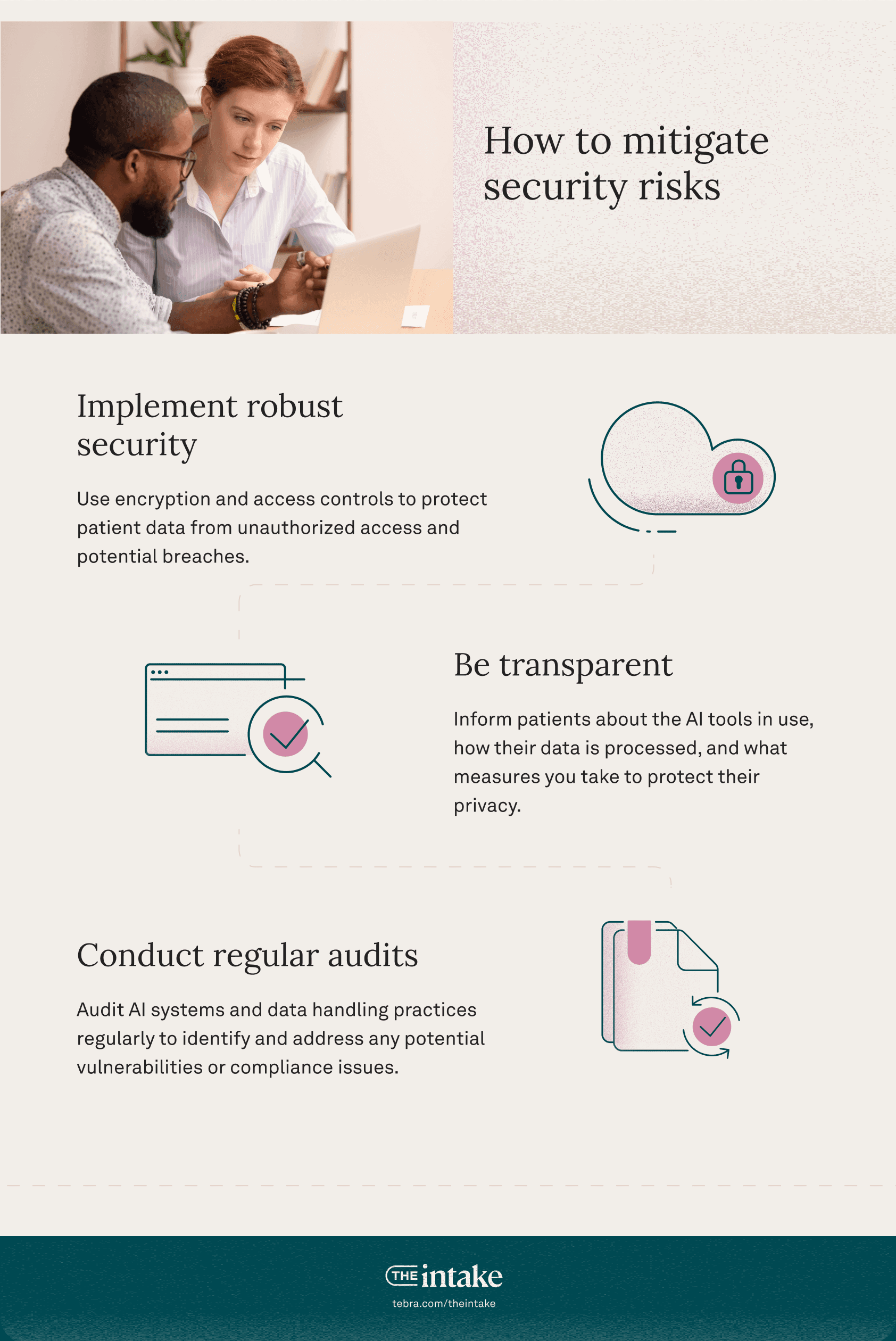
Navigating the future of healthcare AI and HIPAA privacy
As the healthcare industry cautiously adopts artificial intelligence, navigating HIPAA compliance becomes increasingly critical.
“The future of healthcare AI is promising, and offers advancements in diagnosis, treatment, and patient care. However, it is imperative for healthcare providers to rigorously adhere to HIPAA.”
The future of healthcare AI is promising, and offers advancements in diagnosis, treatment, and patient care. However, healthcare providers must rigorously adhere to HIPAA regulations, protecting sensitive patient data while leveraging AI.
This balance is key to maintaining patient trust and the integrity of healthcare services. As technology and regulations evolve, continuous adaptation, education, and ethical considerations will be essential in successfully integrating AI into healthcare practices without compromising on privacy and security.
FAQs
What are the 5 rules of HIPAA?
The 5 main rules of HIPAA are as follows:
- Privacy rule: The federal standard for protecting PHI. These access standards apply to both the healthcare provider and the patient. Example: A practice ensures that only authorized personnel can access a patient's medical records, and patients can request to see their own records or get copies.
- Security rule: The federal standard for managing electronic patient health information. Example: A medical clinic uses encrypted email to send electronic patient health information and secure, password-protected systems to store it.
- Transactions rule: This rule deals with the transactions and code sets used in HIPAA transactions. Example: An insurance company processes healthcare claims electronically using standardized codes and formats specified by HIPAA to ensure consistency and confidentiality in data exchange.
- Unique identifiers rule: Healthcare providers must use a national provider identifier (NPI), a unique 10-digit number, to identify themselves in standard transactions. Example: A general practitioner uses their NPI when submitting electronic claims to ensure a consistent and unique way of identification in all standard transactions.
- Enforcement rule: Establishes guidelines for investigations into HIPAA compliance and enforces the penalties for HIPAA violations. Example: A health regulatory agency investigates a reported HIPAA violation at a dental practice. After determining that the practice improperly disclosed PHI, the agency imposes a fine and mandates a corrective action plan to prevent future breaches.
How is AI used in healthcare data?
Medical settings increasingly use AI in healthcare data management and analysis in several impactful ways:
- Drug development
- Analyzing medical imaging such as x-rays, mammograms, etc.
- Predictive analytics
- Telehealth and remote monitoring
- Supporting patients in virtual wards
- Tracking symptoms and health data to deliver recommended treatment solutions
You Might Also Be Interested In
Optimize your independent practice for growth. Get actionable strategies to create a superior patient experience, retain patients, and support your staff while growing your medical practice sustainably and profitably.
Stay Ahead with Expert Healthcare & Billing Insights
Get the latest industry updates, financial tips, and expert strategies — delivered straight to your inbox.
Suggested for you
Stay Ahead with Expert Healthcare & Billing Insights
Get the latest industry updates, financial tips, and expert strategies — delivered straight to your inbox.