Understanding AI: A medical biller’s guide to essential terms
Learn essential AI terms for medical billing to evaluate software, improve claim accuracy, and boost revenue cycle efficiency.
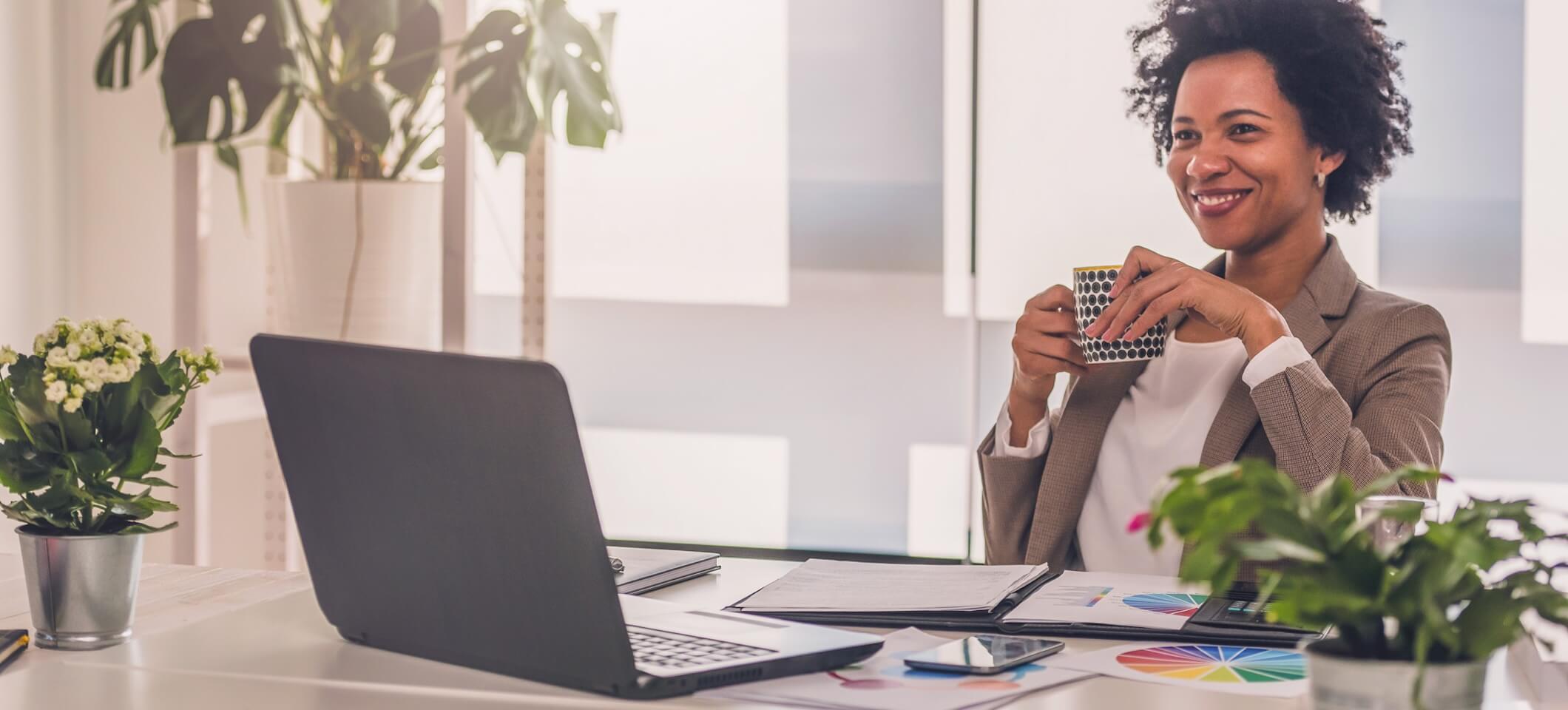
Most Popular
At a Glance
- AI billing tools cut denials with predictive analytics and machine learning.
- NLP extracts diagnosis codes from notes, reducing errors and boosting reimbursements.
- Knowing AI terms helps billers choose the right tech, spot hype, and work smarter — without requiring technical expertise.
Artificial intelligence (AI) is transforming medical billing by making its processes significantly faster and more accurate. You’ve likely heard software vendors describe their solutions as “AI-powered” or “AI-driven.” But to properly assess these tools and communicate their value effectively to your clients, you need to understand the key concepts behind this technology.
This guide breaks down the most important AI terms and gives real-world context by explaining how each concept applies directly to medical billing.
Click on any term below to jump to its section.
- Machine learning (ML)
- Deep learning
- Natural language processing (NLP)
- Robotic process automation (RPA)
- Predictive analytics
- Computer vision
- Supervised learning
- Unsupervised learning
- Algorithm
- Big data
- Data mining
- API (application programming interface)
- Interoperability
- OCR (optical character recognition)
- Bias in AI
What is artificial intelligence (AI) in medical billing?
Definition: AI refers to the ability of computers or software to mimic human intelligence. This includes performing tasks like learning from experience, reasoning, recognizing patterns, and problem solving — things that typically require human brainpower.
“AI refers to the ability of computers or software to mimic human intelligence.”
Relevance: Many of today’s medical billing software and platforms rely on some form of AI. These types of software help streamline tasks like coding, claim submission, denial management, and revenue prediction. Understanding AI allows you to assess whether a vendor’s “AI feature” really adds value, as well as the nature of that value.
Example: Some billing platforms use AI to auto-suggest the most appropriate Current Procedural Terminology (CPT) codes based on chart data or to identify claims likely to be rejected.
Machine learning (ML)
Definition: ML is a form of AI that improves as it sees more data. Think of it as software that gets smarter with experience. It identifies patterns in historical data and uses those patterns to predict outcomes or suggest actions.
Relevance: ML is used in systems that predict claim denials, flag incorrect codes, or optimize revenue cycle performance. For instance, if a payer consistently denies claims when a specific modifier is missing, an ML system can learn that and alert billers automatically. Or if your software starts warning you that a certain payer often denies claims for a specific CPT code combo, that’s likely ML at work.
Scenario: A biller uploads 10,000 historical claims. The ML model identifies trends in denied claims and begins flagging new claims that share those characteristics before they are submitted.
Deep learning
Definition: Deep learning is a subset of machine learning that uses layered algorithms called neural networks. These networks mimic the way the human brain processes information. The more data they process, the better they get at recognizing complex patterns.
Relevance: Deep learning largely does well at understanding unstructured data, like handwritten physician notes or scanned explanation of benefits (EOBs), though current capabilities are still somewhat limited.
Example: Software using deep learning can extract relevant information from .pdfs, images, or free-text fields — saving billers time and reducing manual entry errors.
Natural language processing (NLP)
Definition: NLP is the branch of AI that understands, interprets, and generates human language. It helps to convert speech or text into data with which computers can work.
Relevance: NLP allows software to read and interpret physician notes, helping with code selection, charge capture, and documentation audits.
Example: If your software can pull out relevant diagnosis codes from a paragraph of clinical notes, NLP is doing the heavy lifting.
Robotic process automation (RPA)
Definition: RPA uses software bots to perform repetitive tasks by mimicking human actions within digital systems. These bots click, type, and navigate just like a human user — but they never tire or make typos.
Relevance: RPA can submit claims, post payments, check eligibility, and handle other time-consuming, manual workflows. This frees staff to focus on tasks that require human judgment and strengths, such as resolving rejections or working with patients.
Example: A small billing company uses RPA bots to check patient insurance eligibility across 20 payers every morning. What used to take 2 hours now takes 10 minutes.
Tebra can help you speed up payments, scale growth, and maximize revenue — for you and your clients. Learn more. |
Predictive analytics
Definition: Predictive analytics combines statistics, data modeling, and ML to forecast future outcomes. It uses past data to answer the question, “What’s likely to happen next?”
Relevance: Predictive tools can identify claims likely to be denied, payers that are slower to reimburse, or seasonal dips in cash flow. This insight helps billing teams prioritize work better.
Example: If your platform flags claims with a high risk of denial based on payer trends and patient profiles, it’s using predictive analytics.
Computer vision
Definition: Computer vision is AI that can “see” and interpret visual data, like images or scanned documents or handwriting.
Relevance: Computer vision technology supports automated document processing. It helps read paper forms, scan faxed records, or digitize handwritten physician notes. This eliminates the need to retype information manually.
Example: A billing office uses computer vision to automatically extract payment information from paper EOBs, reducing manual data entry time while improving accuracy. The system can recognize patient details, CPT codes, and payment amounts, even when forms vary or contain handwritten notes.
Supervised learning
Definition: A type of machine learning where the system learns from labeled data that already includes the correct answer, such as from a claim labeled “approved” or “denied.”
Relevance: Supervised learning models can learn from past billing outcomes to predict how future claims will be handled. This helps billing teams proactively adjust submissions for better success rates.
Example: A supervised learning model learns that certain diagnosis-procedure pairs are routinely denied by Medicare and flags them before submission.
Unsupervised learning
Definition: Unsupervised learning is a type of machine learning that finds patterns without being told what to look for. It identifies hidden patterns, groupings, or trends.
Relevance: This can help uncover trends you didn’t even know to look for like unusual payer behavior or missed billing opportunities.
Example: A billing company uses unsupervised learning to analyze thousands of claims and discovers that certain providers consistently underbill for specific procedures compared to their peers. The system automatically identified this revenue opportunity without being explicitly programmed to look for undercoding patterns.
Algorithm
Definition: Algorithms are computational procedures that process data and solve problems. In AI, these range from simple rule-based procedures to complex mathematical models that learn patterns from data.
Relevance: Every AI feature in a billing tool relies on an algorithm working behind the scenes. Some are straightforward (like if-then rules for claim validation) while others are sophisticated (like neural networks that predict denial patterns).
Tip: When evaluating billing software, ask vendors specific questions about their algorithms: How were they developed? What data trained them? How often are they updated? How transparent are their decision-making processes? Understanding these aspects helps you assess whether the tool will perform reliably with your specific billing scenarios.
Big data
Definition: Big data refers to extremely large and complex datasets that traditional technology can't easily process. AI is often used to make sense of this data.
Relevance: Big data allows billing platforms to spot industry-wide trends, benchmark performance, or find coding opportunities across thousands of cases.
Example: A billing company compares its denial rates to a big dataset of 2 million claims to uncover areas for improvement.
Data mining
Definition: The process of AI digging through big data to find useful information. It involves analyzing the data for trends, outliers, and relationships.
Relevance: Data mining can reveal patterns in denial reasons, undercoded visits, or payer behaviors. This informs better decision-making and training at your medical billing practice.
Example: A billing manager uses data mining to identify that one payer denies level 3 office visits 15% more often when billed with a specific diagnosis.
Training data
Definition: The initial dataset used to “teach” an AI or machine learning model how to make decisions.
Relevance: The quality and diversity of training data influences how accurate and fair an AI-based tool will be. If a claims denial prediction tool was trained on claims from only one specialty, it might not work well for others.
Example: A billing tool trained only on cardiology claims may incorrectly flag dermatology claims due to unfamiliar coding patterns.
Application programming interface (API)
Definition: An API is a set of digital rules that let different software systems communicate and share information. Think of it like a digital translator that enables one application to talk to another, requesting or sending data in a standardized format. APIs act as bridges between systems, helping them exchange data seamlessly without needing to know the inner workings of each other.
Relevance: APIs let AI tools integrate with your billing software, electronic health record (EHR) system, or clearinghouses. This allows for seamless data flow, reducing manual entry and errors.
Example: APIs can enable your billing platform to retrieve patient demographics from an EHR or push claim status updates back to a client portal without any manual input on your end.
Interoperability
Definition: Interoperability means that different software systems can exchange and use information in a meaningful way.
Relevance: Without interoperability, your billing software can’t “talk” to other AI tools, client EHRs, or clearinghouses. Interoperability ensures all these different platforms work smoothly together.
Example: A billing company uses interoperable software that integrates with 5 different EHRs, cutting onboarding time for new clients by 50%.
Optical character recognition (OCR)
Definition: OCR is a technology that reads printed or handwritten text and converts it into digital, machine-readable content.
Relevance: OCR is often the first step in digitizing paperwork — paper claims, referrals, EOBs — for AI tools to process. Think of it as the bridge between paper and software.
Example: OCR software reads 100 paper claims an hour and imports them into your billing platform — no manual typing required.
Bias in AI
Definition: AI bias happens when AI systems produce unfair, prejudiced, or preferential outcomes due to flaws in data collection, algorithm design, or implementation. Even systems that seem “neutral” can perpetuate or amplify existing biases.
Relevance: Biased AI in medical billing could disproportionately flag claims from certain provider types, specialties, or patient demographics. This could lead to inequitable processing speeds, reimbursement rates, or audit frequencies. Recognizing potential bias helps ensure your practice uses AI tools that treat all claims and patients equitably.
Tip: Ask vendors about their bias detection and mitigation strategies. Request information about the diversity of their training data and how they test for fairness across different medical specialties, practice sizes, and patient populations.
Embracing AI in medical billing
Understanding these terms will give you the confidence to evaluate new billing software, question vendor claims, and successfully lead your team through digital upgrades. AI isn’t magic — it’s just another tool, and like any tool, its value depends on how well you understand and apply it.
“AI isn’t magic — it’s just another tool, and like any tool, its value depends on how well you understand and apply it.”
Your billing practice should also stay current through webinars, vendor demos, and industry associations. The more you and your team understand how AI works, the better you can use it to serve your clients and boost your bottom line.
You Might Also Be Interested In
Medical billing company best practices: Learn why diversifying, digitizing, and operational transparency are your keys to growth in our benchmark report.
Stay Ahead with Expert Healthcare & Billing Insights
Get the latest industry updates, financial tips, and expert strategies — delivered straight to your inbox.
Suggested for you
Stay Ahead with Expert Healthcare & Billing Insights
Get the latest industry updates, financial tips, and expert strategies — delivered straight to your inbox.